Bittensor’s Decentralized AI Marketplace: An In-Depth Review
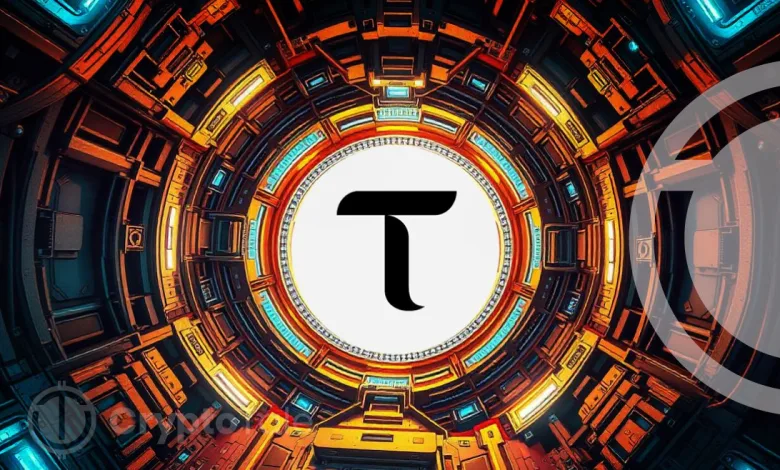
Bittensor is an open-source protocol designed to establish a decentralized machine-learning network. It is designed to transform artificial intelligence (AI) into a tradable commodity and occurs within a transparent marketplace. Further, the AI models are based on comprehensive intelligence and utility. They are not limited to performance metrics.
Traditional AI frameworks are centralized. However, Bittensor encourages collaborative AI development across a decentralized network, prioritizing holistic intelligence. As a result, it fosters innovation and enhances transparency in AI development.
How Bittensor Came to Be
Traditional AI development models forced Bittensor to be created. Traditionally, AI training has gone something like training racehorses. It is focused specifically on some tasks with clearly defined benchmarks. The narrow approach frequently restricts the larger potential of AI. Consequently, it generates generalized solutions as opposed to innovative and specialized outputs.
Small AI models are also marginalized by traditional frameworks. These smaller models could have this unique problem solving capability. But they are often missing, leading to a less inclusive AI space.
To overcome these challenges, Bittensor was developed. It is to decentralize AI training and evaluation. Blockchain technology is used by Bittensor to create a more transparent and fair marketplace. Based on their overall contributions, AI models are assessed and rewarded on an aggregate basis. This also leads into a more inclusive ecosystem. This lets people of all sizes and origins participate and thrive with AI models.
Use Cases of Bittensor’s Decentralized AI
Bittensor offers diverse use cases that reflect its versatile structure. AI researchers and developers can collaborate over decentralized networks. They are enabled to provide to shared AI models through these networks. The intelligence of the models is quite diverse.
Innovative Solutions for Specific Sectors: Bittensor’s subnet feature enables the creation of sub-networks tailored to specific industries. These industries include finance, gaming, and translation. This allows for the development of customized AI models. These models are then rewarded based on their impact and performance within their domains.
Inclusive AI Participation: Smaller AI developers and independent researchers can access Bittensor’s decentralized network. This allows them to contribute to AI innovation, providing opportunities for experienced developers who lack resources.
Secure and Transparent AI: Transactions Bittensor uses blockchain technology to enhance security and transparency. This ensures all transactions within its AI marketplace are secure, transparent, and immutable.
How Bittensor’s Decentralized Network Works
Bittensor operates as a decentralized network for AI models. They interact within a blockchain-based ecosystem. Structured as a central hub, it connects multiple subnets, representing distinct AI-related tasks or industries.
Currently, there are 32 subnets on Bittensor, each serving a different purpose. Some subnets focus on machine translation. Others handle natural language processing (NLP), gaming, or financial forecasting.
The Subnets: Specialized Communities of AI
Each subnet in Bittensor serves as an independent community with a distinct purpose. Participants can create or join subnets by paying a registration fee in TAO tokens, Bittensor’s native cryptocurrency. Subnet members can act as either miners or validators:
Miners contribute to completing assigned tasks within a subnet. They generate responses to text prompts or conduct machine translations.
Validators assess the performance of miners, ensuring that the outputs are accurate and of high quality. Validators then reward miners accordingly, incentivizing productive behavior and enhancing overall subnet performance.
Subnets operate using Bittensor’s blockchain platform, subtensor. It documents activities, ensuring transparency, accountability, and proper rewards. The Yuma Consensus mechanism supports the network. It calculates the blockchain rewards every 12 seconds, based on the contributions of miners and validators. This system encourages honest behavior by rewarding validators for accurate and truthful reports.
Incentive Mechanisms and Governance
Bittensor’s decentralized AI ecosystem also incorporates dynamic incentive mechanisms. It aligns with the learning paradigms of its subnets. Validators use a weight matrix to highlight the prominent areas of knowledge. This prompts miners to produce outputs that reflect these areas. This competitive dynamic encourages rapid innovation and diversified AI outputs.
The platform’s governance is decentralized and there is no one controls the network. This ensures that AI development within Bittensor is open and fair. This will enable all contributors to influence decision-making and steer network growth.
Advantages of Bittensor’s Decentralized AI Model
Bittensor’s decentralized AI model offers several key benefits:
Enhanced Privacy and Security: It eliminates large-scale breaches. Data is distributed across multiple nodes. This ensures robust data protection.
Resilience to Failures: Bittensor’s decentralized structure limits the failures of individual nodes, ensuring consistency in output and operations.
Increased Inclusivity: Small-scale developers and individuals could enhance the landscape of AI.
Transparent Transactions: Blockchain technology in Bittensor ensures that all transactions and decisions are transparent and immutable. This builds trust among participants.
Dynamic Scalability: Bittensor’s open structure allows for seamless integration of new participants and computational resources, supporting the network’s scalability.
Disadvantages of Decentralized AI in Bittensor
Despite its advantages, Bittensor’s decentralized AI marketplace has a few limitations:
Complexity of Governance: Decentralization promotes fairness. But it hinders governance process. In many cases the deaer makes slower or more fragmented decisions.
Potential for Collusion:There is no room for dishonesty in the Yuma Consensus. However, this doesn’t mean that validators will not collude. For example, such collusion could influence the distribution of rewards.
Higher Learning Curve: Bittensor’s decentralized structure presents unique challenges. Its consensus mechanisms can be difficult to understand. For new users, this creates barriers to entry.
Limited Computational Resources: The network supports scalability. However, some subnets face computational constraints. These limitations can reduce the effectiveness of AI models.
Conclusion
Bittensor introduces a brand new AI development methodology. It moves from centralized task specific frameworks. Here it is a decentralized, collaborative model. It takes a holistic intelligent approach as well as promoting fair incentive. Therefore, it fosters inclusivity, innovation, and transparency at the global scale of AI ecosystem.
With the advancement of Bittensor, it evolves to become a decentralized AI system opposite traditional traditional AI systems. I prefer to say that the platform is push the equity and extensibility. This guarantees that there is fair participation between the AI landscape. As it advances with its decentralized structure, Bittensor improves its incentive mechanisms. They enhance the platform by keeping it front of the pack in the space of AI innovation. In the end it thus serves to promote collaborative intelligence that goes beyond conventional borders.